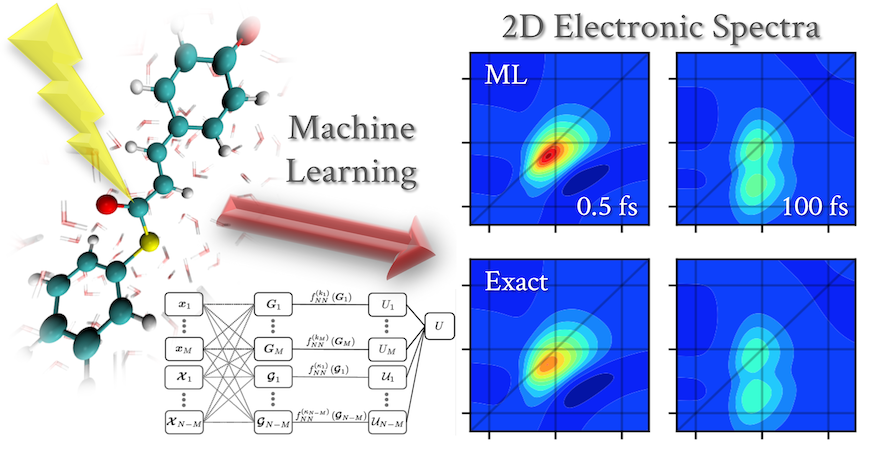
The Isborn group's work published in the Journal of Physical Chemistry Letters uses machine learning frameworks to dramatically accelerate the simulation of multidimensional optical spectra for solvated biologically important chromophores. Traditionally these simulations have been prohibitively expensive, since they require tens of thousands of excited state electronic structure calculations containing both the chromophore and its chemical environment in order to accurately calculate the spectra. The machine learning models trained on only ~1000 such calculations are able to accurately and efficiently predict the energy gaps needed to simulate these optical spectra. This reduction in the number of expensive excited state calculations amounts to a computational savings on the order of a quarter million graphical processing unit (GPU) compute hours. This work thus opens the door to the routine simulation of multidimensional optical spectra of large condensed phase systems using advanced dynamics-based methods that would otherwise be too computationally expensive.